Live
- Lucky foods to kickstart a prosperous New Year
- Naushad: The maestro who revolutionised Indian film music
- APSRTC Announces 1000 Special Bus Services from Vizag for Sankranti
- Hello Hyderabad, get ready for New Year bash
- Lakshya Sen loses in semifinals of King Cup Int’l
- Govardhan, Thanuja triumph at Telangana State Sailing Championships
- HCA chief felicitates U-19 cricketers Trisha, Drithi
- 2 held for killing fake kidnap mastermind
- ISL: Hyderabad FC score late to deny East Bengal full points
- Reddy’s maiden ton keeps India afloat
Just In
AI-powered transformation in manufacturing: Balachandar Ramalingam's vision for component management excellence
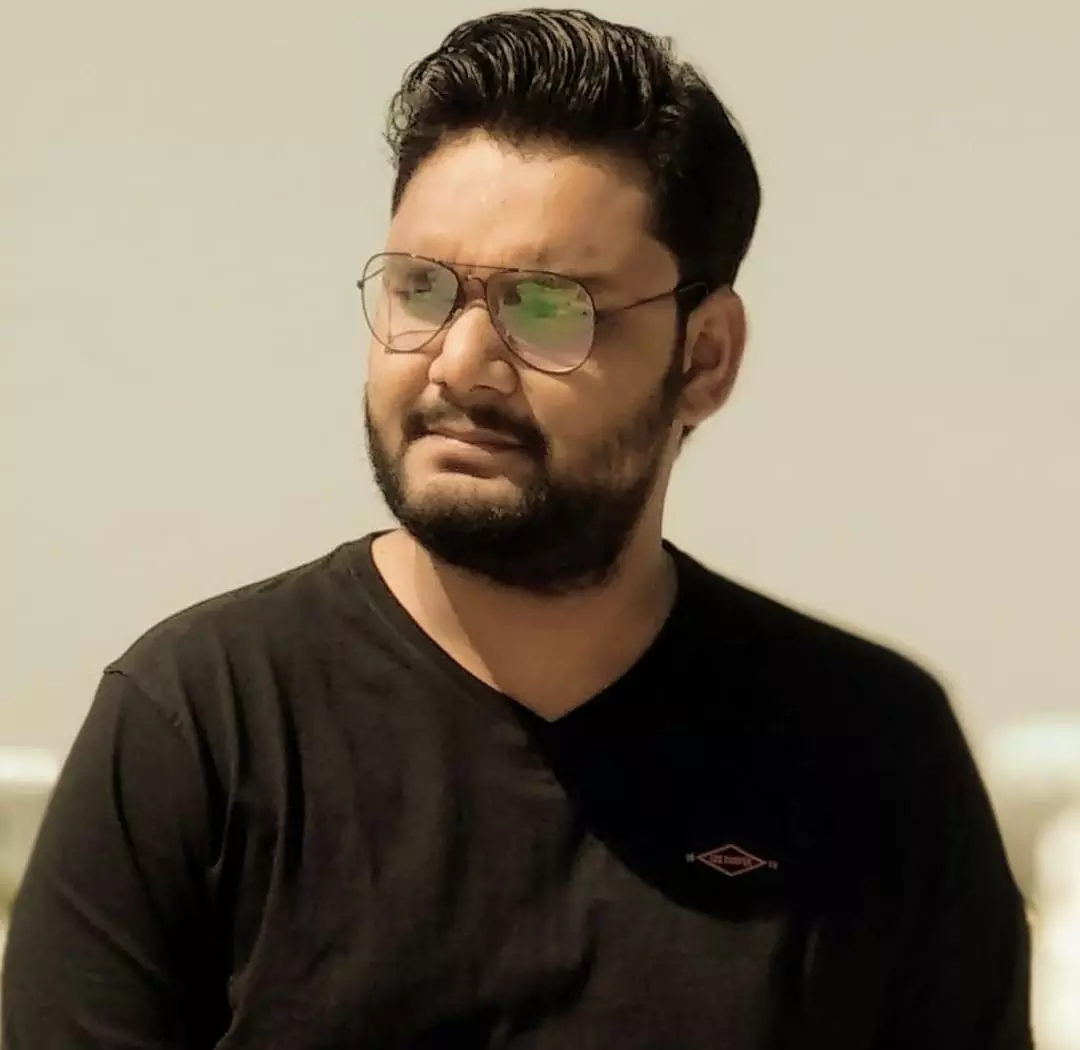
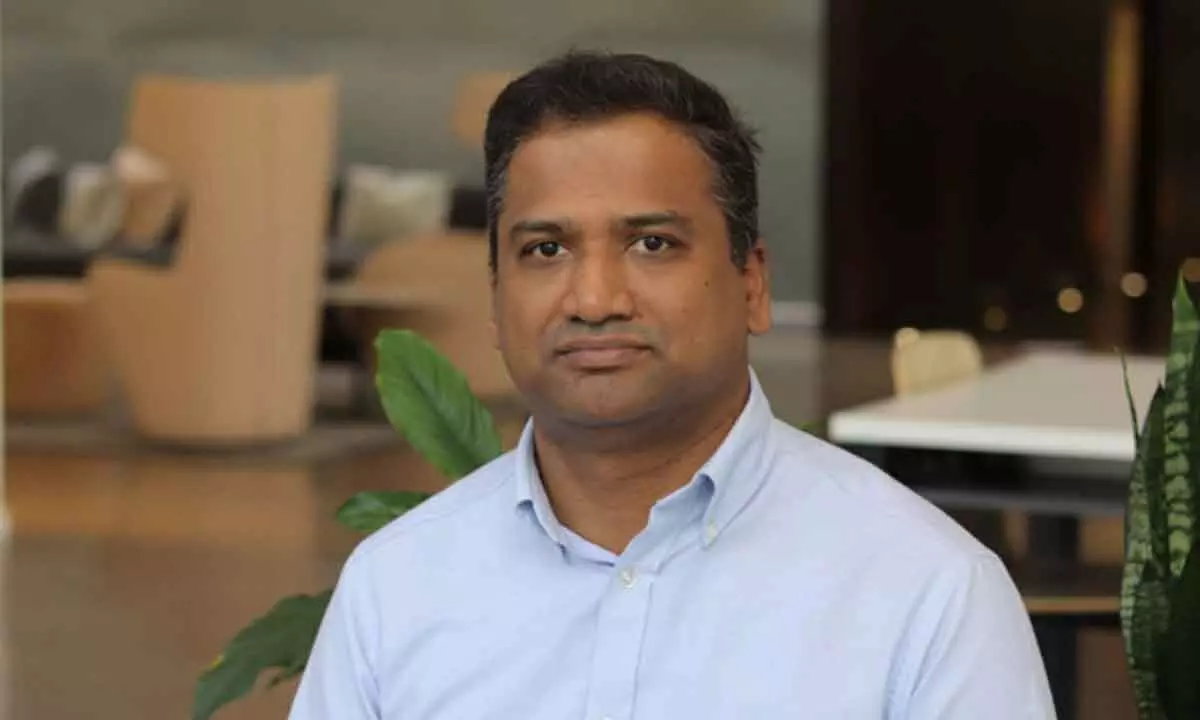
In a rapidly evolving digital landscape, Balachandar Ramalingam's leadership in implementing an AI-driven 3D Data Classification and Standardization System marks a groundbreaking achievement in component management for global product lines.
In a rapidly evolving digital landscape, Balachandar Ramalingam's leadership in implementing an AI-driven 3D Data Classification and Standardization System marks a groundbreaking achievement in component management for global product lines. In this interview, we discuss the challenges, innovations, and significant impact of this project, which has redefined intelligent manufacturing processes.
Could you start by telling us what motivated you to pursue this AI-based transformation in component management?
Certainly! The motivation stemmed from the complexities of managing increasingly diverse product portfolios. As operations expanded globally, legacy systems struggled to keep pace with the demand for accurate component identification. This led to inefficiencies, higher inventory costs, and longer procurement cycles. We needed a solution that could automate and standardize the way we classified and managed components, and AI was the perfect fit for this challenge.
What was the core idea behind the AI-based system you developed?
The core idea was to build an intelligent, shape-based component classification system powered by AI. We focused on creating a centralized repository of standardized component data, accessible across all our global operations. The AI model we developed could automatically identify, classify, and match components based on their shapes, ensuring consistency and reducing redundancies. This system also made it easier for teams to quickly find and reuse components across different product lines, which was a game-changer for us.
How did the collaboration with cross-functional teams play a role in the success of this project?
Collaboration was crucial. We worked closely with data scientists, design engineers, supply chain teams, and manufacturing experts. Each team brought unique insights into the classification process and its challenges. This cross-functional collaboration helped refine the AI model and ensure its successful implementation across various regions. Having a unified approach allowed us to address complex problems more effectively and align our efforts with the global strategy.
What were the measurable outcomes of this initiative?
The impact was substantial. We achieved a 20% reduction in inventory costs through improved component identification and reuse. Our supply chain efficiency also increased by 18%, thanks to better component standardization and streamlined procurement processes. Most importantly, overall profitability improved by 10%, which highlights the broader operational benefits of the system. The project didn’t just save costs – it also optimized our resources and accelerated product development cycles.
How did this project improve time-to-market and resource utilization?
By reducing procurement lead times and enabling teams to reuse components more efficiently, we could get products to market much faster. The AI system also helped identify underutilized components across product lines, improving resource allocation. This meant that manufacturing teams could focus on innovation instead of spending excessive time sourcing and managing new parts.
What were some key insights you gained from this transformation process?
One key insight was the importance of having high-quality training data for machine learning models. The accuracy of the system depended heavily on the data we fed it. Another important lesson was the need for continuous improvement – the AI model had to be constantly refined based on real-world feedback. We also learned that proper integration and alignment across teams were essential for successful system adoption.
Looking ahead, what does the future hold for AI in manufacturing?
This project has set a strong foundation for future AI applications in manufacturing. The success we’ve seen with component classification can be extended to other areas like predictive maintenance and supply chain optimization. I believe AI will continue to revolutionize manufacturing by improving efficiency, reducing costs, and driving innovation.
Finally, how has this project impacted your career?
This project has been a significant milestone in my career. It not only deepened my expertise in AI but also gave me invaluable experience in leading large-scale systems deployment. The success of this initiative has positioned me as an innovator in manufacturing technology, and I’m excited to continue exploring new ways AI can enhance industrial processes.

© 2024 Hyderabad Media House Limited/The Hans India. All rights reserved. Powered by hocalwire.com