Live
- YSRCP blamed for delay in bypass road completion
- Minister discusses ‘loan for capital’ with Hudco CMD
- BJP highly respects Ambedkar: Purandeswari
- Sensex, Nifty edge down in sluggish trading
- All-round development of CM native village on anvil
- Economy gets into recovery mode after Q2 slowdown
- Fieo charts strategy to push exports to US
- AP to receive rains today and tomorrow amid severe low pressure
- Job scam: No High Court bail for Partha
- Joint teams from AP, TG destroy illicit liquor
Just In
Rahul Vadisetty: Harnessing AI to revolutionize predictive healthcare
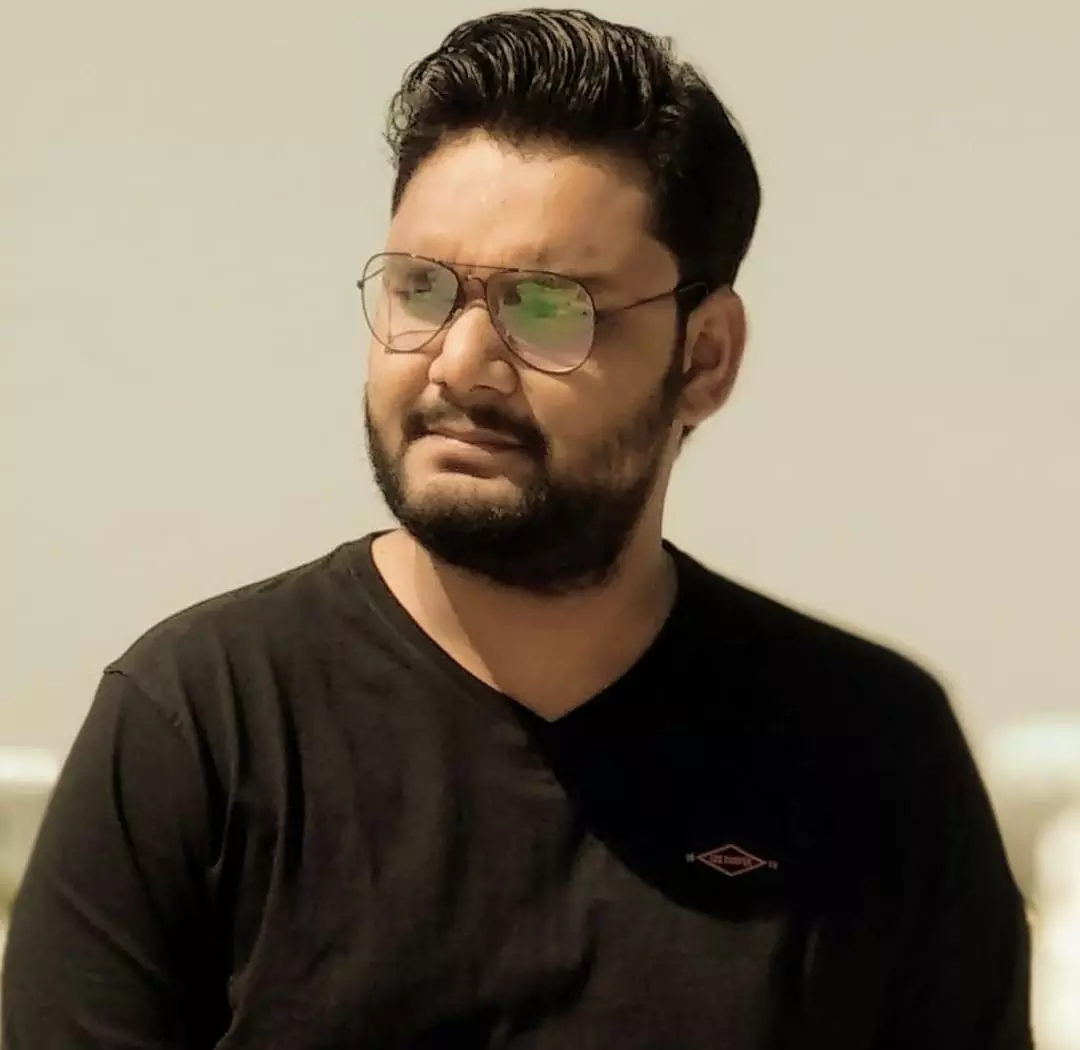
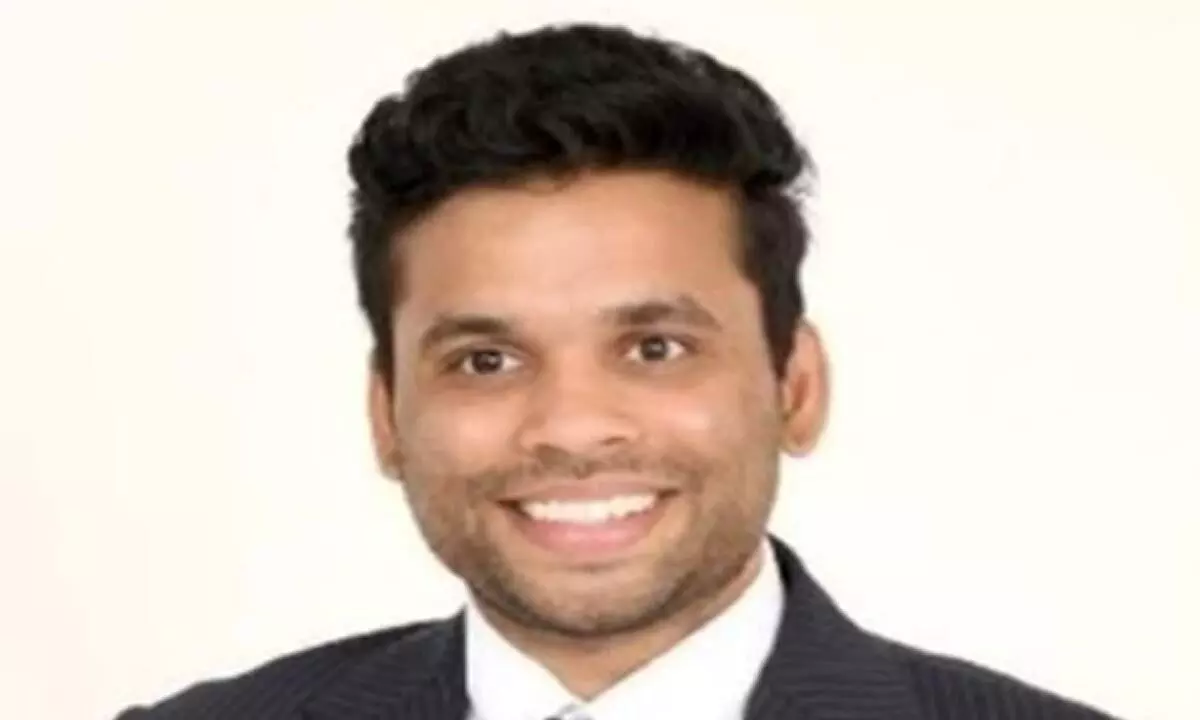
In the realm of healthcare innovation, few have made as significant an impact as Rahul Vadisetty. His groundbreaking research, “Advancing Predictive Modelling in Healthcare: A Data Science Approach Utilizing AI-Driven Algorithms,” recently won the Best Paper Award at the IEEE conference.
In the realm of healthcare innovation, few have made as significant an impact as Rahul Vadisetty. His groundbreaking research, “Advancing Predictive Modelling in Healthcare: A Data Science Approach Utilizing AI-Driven Algorithms,” recently won the Best Paper Award at the IEEE conference. His work demonstrates how AI can transition healthcare from reactive to proactive, enabling earlier disease detection and improving patient outcomes. In this interview, we explore Vadisetty’s insights on AI in healthcare, predictive modelling, and the future of data-driven medicine.
Rahul, your research has gained a lot of attention in the healthcare space. Can you tell us more about the core focus of your work?
At its core, my research is focused on using predictive analytics to drive better healthcare outcomes. By applying AI-driven algorithms, we’re working to move from reactive healthcare—where we respond to illnesses after they occur—to proactive healthcare, where we can predict and prevent disease before it becomes critical. One of the key features of this work is the use of Explainable AI (XAI), which aims to make AI models more transparent and interpretable, something that’s crucial in clinical settings where trust and accountability are paramount.
Explainable AI sounds like a significant innovation. How does this work in healthcare?
Traditional AI models often operate as "black boxes," making decisions without providing clear explanations for their predictions. In healthcare, this lack of transparency can be a barrier to adoption. With Explainable AI, we ensure that clinicians can understand why an AI model has made a specific recommendation, allowing them to trust the results and make better-informed decisions. For example, in chronic disease management, such as diabetes or heart disease, AI can predict disease progression, but clinicians must be able to understand why certain treatments or interventions are recommended.
Speaking of chronic diseases, how does your research address challenges in managing conditions like diabetes or heart disease?
Chronic diseases are among the biggest healthcare challenges globally. My research focuses on applying AI to predict the progression of these diseases and recommend personalized treatment plans. For diabetes, for instance, we use Reinforcement Learning (RL) algorithms to optimize long-term treatment strategies, analyzing patient data to recommend the best combinations of medications and lifestyle changes. This proactive approach can reduce complications and improve patient outcomes, ultimately lowering healthcare costs.
Beyond patient care, how does your work impact operational efficiency in healthcare systems?
AI can also significantly improve the operational efficiency of healthcare organizations. By predicting patient admissions, optimizing resource allocation, and reducing hospital readmission rates, AI can streamline operations and save costs. For example, predictive models can identify patients at high risk of readmission, allowing healthcare providers to intervene earlier, improving outcomes and reducing the strain on healthcare resources. AI is also vital in supply chain management, ensuring that pharmaceuticals and medical supplies are delivered on time and in the right quantities.
What ethical considerations do you think are essential in AI’s integration into healthcare?
Ethical AI is critical in healthcare, where patient safety and privacy are paramount. We must ensure that AI systems are transparent, accountable, and unbiased. My research emphasizes the need for models that are not only accurate but also fair, addressing potential biases that could affect marginalized groups. Ensuring that AI systems respect patient data and provide equitable care is key to maintaining trust and achieving the full potential of AI in healthcare.
What’s next for you in terms of AI research and healthcare innovation?
Moving forward, I am particularly excited about the scalability of AI models and their integration with real-time data from wearable devices and electronic health records. These technologies will create a more personalized and responsive healthcare system. My focus will be on refining predictive models to address healthcare disparities and ensuring that AI can offer real-time, actionable insights for better patient care and more efficient healthcare delivery.

© 2024 Hyderabad Media House Limited/The Hans India. All rights reserved. Powered by hocalwire.com