Live
- ISL: Hyderabad FC score late to deny East Bengal full points
- Reddy’s maiden ton keeps India afloat
- Gold rates in Delhi today stable, check the rates on 28 December, 2024
- ‘A knock to remember’: Tendulkar hails Nitish Reddy’s maiden Test century
- BJP accuses Rahul of indulging in politics
- Reliance’s CSR move
- BJD urges State govt to waive farm loans
- State Law Commission formed
- KLH celebrates achievements
- Hindustan Zinc’s initiative
Just In
Revolutionizing marketplace pricing with Machine Learning: The strategic innovation of Abhijeet Bajaj
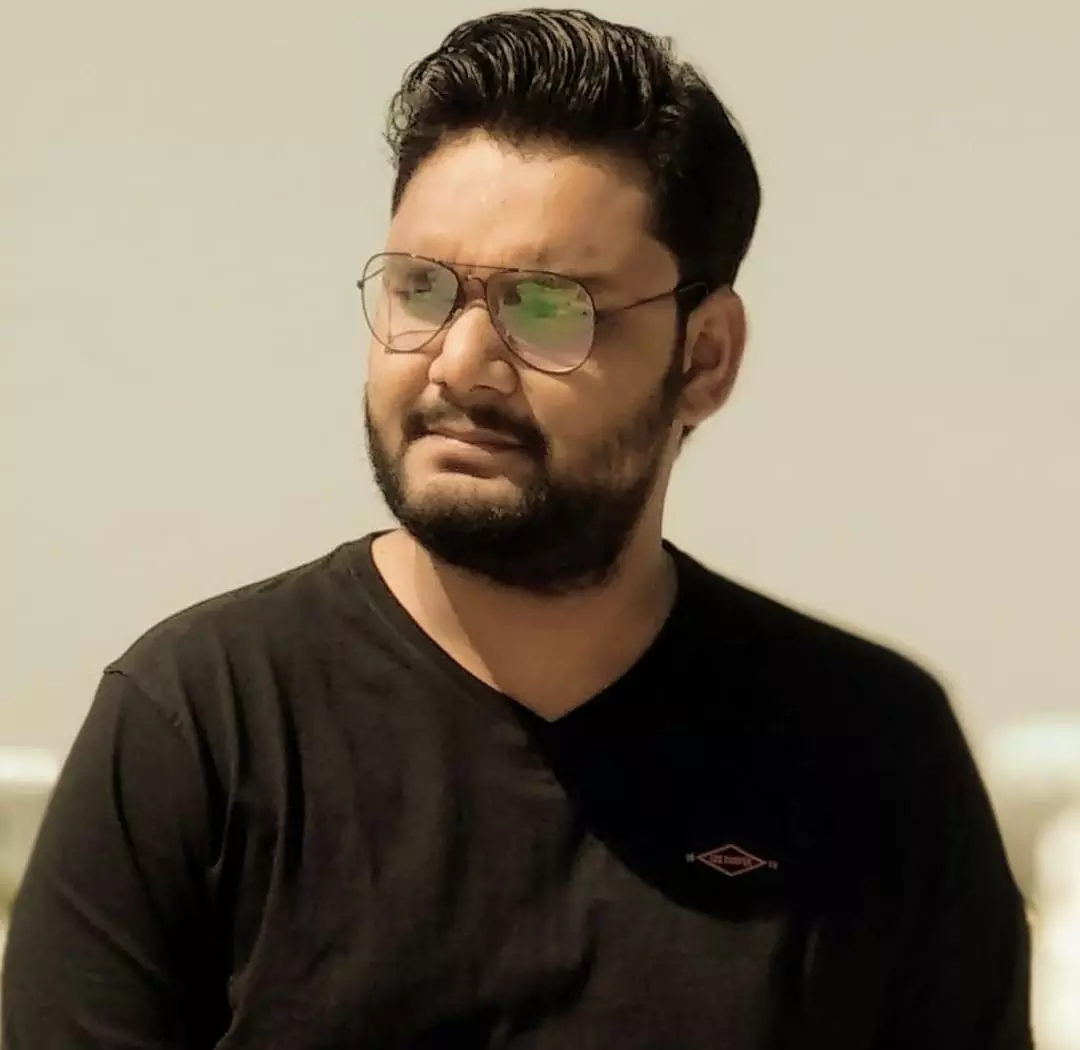
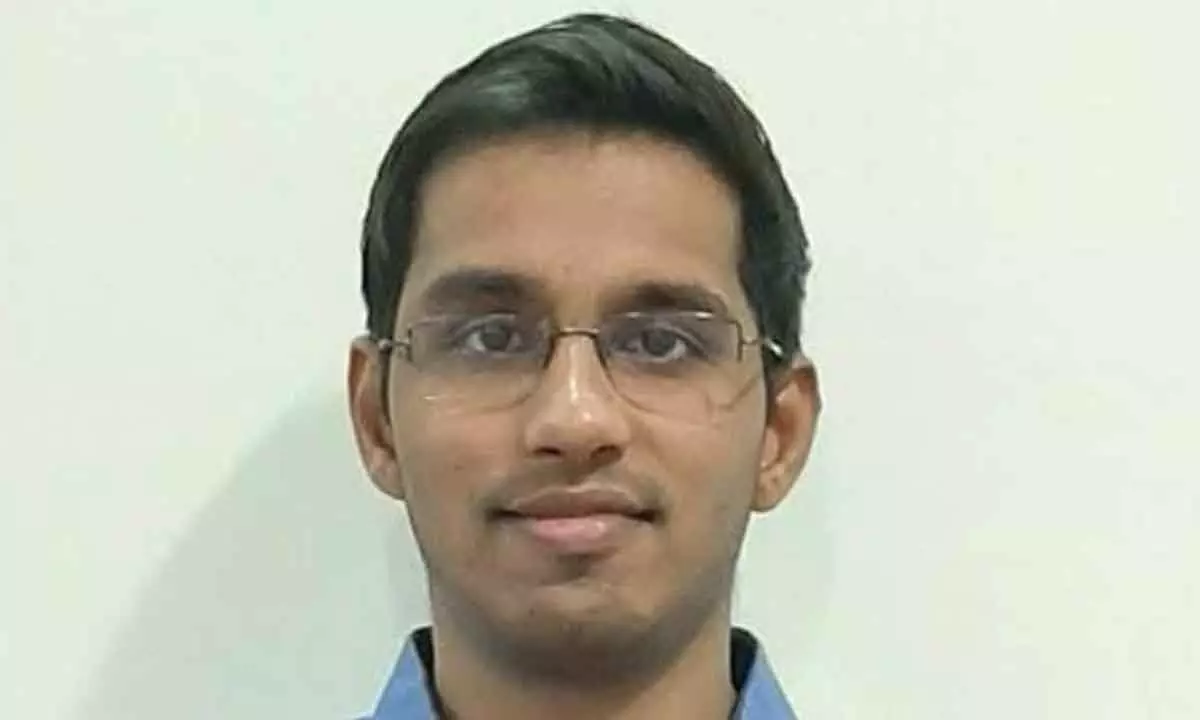
In the ever-evolving world of digital marketplaces, managing the delicate balance of supply and demand is a constant challenge. Abhijeet Bajaj, a Software Engineer, led a pivotal project aimed at optimizing dynamic pricing for Latin American markets using machine learning.
In the ever-evolving world of digital marketplaces, managing the delicate balance of supply and demand is a constant challenge. Abhijeet Bajaj, a Software Engineer, led a pivotal project aimed at optimizing dynamic pricing for Latin American markets using machine learning. In this interview, Bajaj shares insights into how advanced algorithms and collaborative efforts resulted in improved marketplace efficiency.
Can you tell us about the project you led that focused on dynamic pricing for Latin American markets?
Certainly! The project was designed to optimize real-time supply and demand matching in Latin America. The core objective was to predict undersupply conditions and manage a $1.5 billion annual incentive budget efficiently. To achieve this, we needed to integrate machine learning techniques to improve prediction accuracy and enhance real-time monitoring.
What were the two main goals you aimed to achieve with this initiative?
The primary goals were to accurately predict undersupply situations and apply targeted incentives to balance supply and demand. The second objective was to optimize the allocation of our $1.5 billion annual budget, ensuring we could provide effective incentives without overspending.
How did you and your team approach the problem?
We collaborated closely with data scientists and engineers to create a sophisticated pricing algorithm. This algorithm was designed to predict real-time supply shortages and adjust pricing strategies accordingly. We applied machine learning to enhance prediction accuracy and incorporated advanced monitoring systems that provided insights into various marketplace conditions.
What role did collaboration play in the success of this project?
Collaboration was key. We worked with cross-functional teams to refine the algorithm, ensuring it met business needs. For example, engineers helped optimize the algorithm’s performance, while data scientists ensured we were using the most effective machine learning models. This seamless integration across teams made the project successful.
What measurable improvements did you see in the marketplace as a result of the algorithm?
We saw significant improvements in several areas. Our advanced monitoring dashboards allowed us to track key metrics like trip recall in surging areas, and we could precisely measure intersections over unions. The real-time data tracking and optimized pricing led to improved supply-demand matching, making the marketplace more stable and efficient.
How did this project impact the financial side of the business?
Financially, we managed the $1.5 billion incentive budget efficiently, ensuring that the funding was allocated properly to maintain marketplace stability. By improving the accuracy of our predictions and dynamically adjusting pricing, we were able to deliver smoother operations and generate substantial operational benefits. The cost-efficiency was definitely a highlight.
Beyond operational improvements, what broader lessons did you learn from this project?
One of the biggest takeaways was the importance of high-quality data in machine learning applications. There’s a fine balance between accuracy and operational efficiency. We also learned that cross-functional integration is crucial for the success of any large-scale project.
What’s next for marketplace optimization, and how does this project pave the way for future developments?
This project sets the foundation for further advancements in marketplace optimization. By using machine learning to predict supply-demand mismatches, we’ve opened the door for more efficient resource allocation and better business outcomes. The insights gained will drive future innovations in dynamic pricing across various markets.
On a personal note, how has this project shaped your career and expertise?
It has been a milestone in my career. I’ve gained deeper expertise in machine learning, real-time systems, and advanced analytics in digital marketplaces. The experience of deploying complex algorithms and optimizing large-scale systems has strengthened my foundation in marketplace optimization.
Abhijeet Bajaj’s innovative approach to dynamic pricing and machine learning has not only enhanced operational efficiency but has also set a new standard for marketplace optimization. His project stands as a testament to the power of data-driven solutions in solving complex real-world challenges.

© 2024 Hyderabad Media House Limited/The Hans India. All rights reserved. Powered by hocalwire.com