Live
- Govt awaits Governor's nod to prosecute KTR
- CM exhibits ignorance with his statements on Guv: Kishan
- Amid buzz over arrest, KTR says he is ready to go to jail
- Govt trying to grab lands for a song: BJP MP
- BRS an obstacle for investments: Jagga Reddy
- TGPSC all set for holding Group-III exams
- Delays dog LRS cases as applications pile-up
- TGSPDCL deploys 101 ERT vehicles
- JIH to host national conclave in city
- OU celebrates National Library Week with book exhibition
Just In
MathWorks Announces Release 2017b of the MATLAB and Simulink Product Families
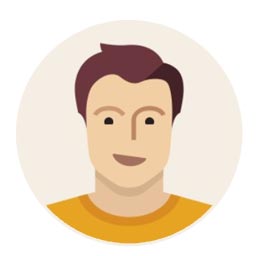
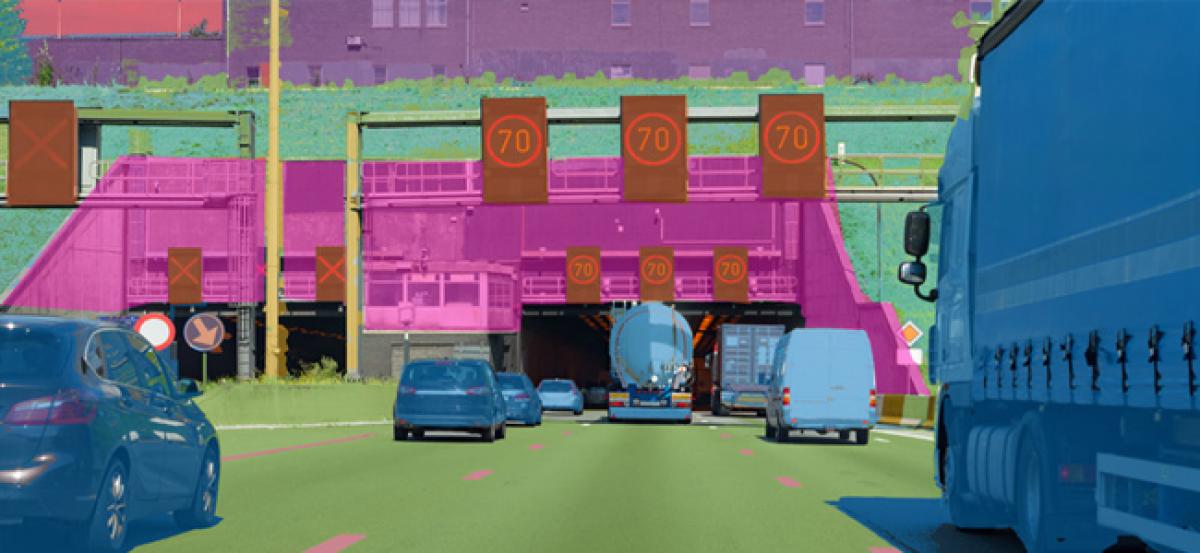
MathWorks today introduced Release 2017b (R2017b), which includes new features in MATLAB and Simulink, six new products, and updates and bug fixes to 86 other products. The release also adds new important deep learning capabilities that simplify how engineers, researchers, and other domain experts design, train, and deploy models.
Strengthens Deep Learning capabilities to simplify designing, training, and deploying models
India, Bangalore: MathWorks today introduced Release 2017b (R2017b), which includes new features in MATLAB and Simulink, six new products, and updates and bug fixes to 86 other products. The release also adds new important deep learning capabilities that simplify how engineers, researchers, and other domain experts design, train, and deploy models.
Deep Learning Support
Specific deep learning features, products, and capabilities in R2017b include:
· Neural Network Toolbox has added support for complex architectures, including directed acyclic graph (DAG) and long short-term memory (LSTM) networks, and provides access to popular pretrained models such as GoogLeNet.
· The Image Labeler app in Computer Vision System Toolbox now provides a convenient and interactive way to label ground truth data in a sequence of images. In addition to object detection workflows, the toolbox now also supports semantic segmentation using deep learning to classify pixel regions in images and to evaluate and visualize segmentation results.
· A new product, GPU Coder, automatically converts deep learning models to CUDA code for NVIDIA GPUs. Internal benchmarks show the generated code for deep learning inference achieves up to 7x better performance than TensorFlow and 4.5x better performance than Caffe2 for deployed models.*
Together with capabilities introduced in R2017a, pretrained models can be used for transfer learning, including convolutional neural networks (CNN) models (AlexNet, VGG-16, and VGG-19), as well as models from Caffe (including Caffe Model Zoo). Models can be developed from scratch, including using CNNs for image classification, object detection, regression, and more.
“With the growth of smart devices and IOT, design teams face the challenge of creating more intelligent products and applications by either developing deep learning skills themselves, or relying on other teams with deep learning expertise who may not understand the application context,” said David Rich, MATLAB marketing director, MathWorks. “With R2017b, engineering and system integration teams can extend the use of MATLAB for deep learning to better maintain control of the entire design process and achieve higher-quality designs faster. They can use pretrained networks, collaborate on code and models, and deploy to GPUs and embedded devices. Using MATLAB can improve result quality while reducing model development time by automating ground truth labeling.”

© 2024 Hyderabad Media House Limited/The Hans India. All rights reserved. Powered by hocalwire.com